This blog relates to experiences in the Systems Capacity and Availability areas, focusing on statistical filtering and pattern recognition and BI analysis and reporting techniques (SPC, APC, MASF, 6-SIGMA, SEDS/SETDS and other)
Popular Post
-
I have got the comment on my previous post “ BIRT based Control Chart “ with questions about how actually in BIRT the data are prepared for ...
-
Your are welcome to post to this blog any message related to the Capacity, Performance and/or Availability of computer systems. Just put you...
_
Wednesday, February 7, 2018
More about #CloudCapacityPlanning from #CMGnews
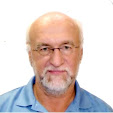
Visiting #CapitalOneCafe
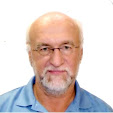
What is Capacity Management?
What is Capacity Management? [Webinar Recap]: Capacity management is the practice of making sure IT resources meet business demands today and down the road—without over-provisioning. But the role of capacity management has changed as IT environments have evolved.
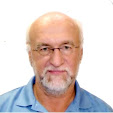
Tuesday, February 6, 2018
"Machine Learning for Predictive Performance Monitoring" - interesting #CMGJournal article (#CMGnews)
Tim Browning has a lot of good publications about Capacity Management in www.CMG.org and also in this blog:
"Entropy-Based Anomaly Detection for SAP z/OS Systems"
#CMGamplify - "#DataScience Tools for Infrastructure
Operational Intelligence"
"the
review of cloud computing article "Optimal Density of Workload Placement"
"Entropy-Based Anomaly Detection for SAP z/OS Systems"
#CMGamplify - "#DataScience Tools for Infrastructure
Operational Intelligence"
"the
review of cloud computing article "Optimal Density of Workload Placement"
He has just published his new paper in the CMG Journal:
"Machine Learning for Predictive Performance Monitoring",
which is available for CMG members
I have enjoyed reading the paper, below is the abstract:
I like especially his following very true saying:
"...Machines don’t actually “learn” nor do statistical algorithms represent some mechanistic disembodied intelligence. However, human learning and intelligence is greatly assisted by statistical modeling in much the same way that optics technology assists vision..."
I appreciate he referenced two my CMG papers under his "Useful Related Materials" section:
- Trubin, Igor, “Exception Based Modeling and Forecasting”, CMG2008 Proceedings
- Trubin, Igor, “Capturing Workload Pathology by Statistical Exception Detection System”,
CMG2005 Proceedings.
I appreciate he referenced two my CMG papers under his "Useful Related Materials" section:
- Trubin, Igor, “Exception Based Modeling and Forecasting”, CMG2008 Proceedings
- Trubin, Igor, “Capturing Workload Pathology by Statistical Exception Detection System”,
CMG2005 Proceedings.
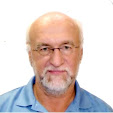
Friday, February 2, 2018
YouTube playlist "My Work"
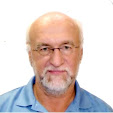
#ROBOTS IN MY LIFE - YT playlist
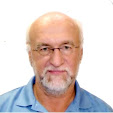
Thursday, February 1, 2018
#AnomalyDetection vs. #NoveltyDetection. SETDS Method Detects and Separates both
Reading "Anomaly detection with Apache MXNet":
"An important distinction has to be made between anomaly detection and “novelty detection.” The latter turns up new, previously unobserved, events that still are acceptable and expected. For example, at some point in time, your credit card statements might start showing baby products, which you’ve never before purchased. Those are new observations not found in the training data, but given the normal changes in consumers’ lives, may be acceptable purchases that should not be marked as anomalies."
I figured out that my SETDS method has this Novelty Detection included as my
"An important distinction has to be made between anomaly detection and “novelty detection.” The latter turns up new, previously unobserved, events that still are acceptable and expected. For example, at some point in time, your credit card statements might start showing baby products, which you’ve never before purchased. Those are new observations not found in the training data, but given the normal changes in consumers’ lives, may be acceptable purchases that should not be marked as anomalies."
I figured out that my SETDS method has this Novelty Detection included as my
EV based trends detection method (e.g. implemented in R as "TrendieR") finds recent change points in the time-serious data and then by building trend-forecast checks if the change is permanent or not. So if it is permanent the possible "novelty" is detected.
So the 1st part of SETDS (e.g. implemented as "SonR" on R) captures just anomalies and/or outliers, then Trend detection separates cases that indicate the possible "novelty". (something changed and stays changed and growing). Still false positive could be there though....
BTW there is a 3rd level of SETDS which is actually the way to correlate performance data with demand (drivers) data to build meaningful forecasts (e.g. implemented as "Model Factory")
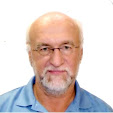
Subscribe to:
Posts (Atom)